Multimodal image fusion system for brain tumor segmentation
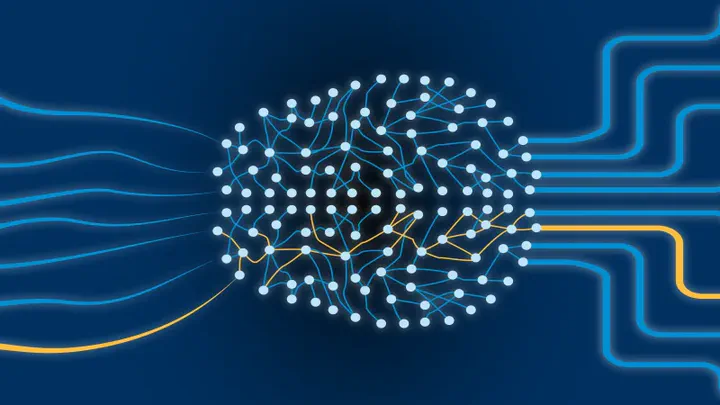
Overview
Multimodal Image Fusion System for Brain Tumor Segmentation is a project dedicated to improving the accuracy and efficiency of brain tumor classification and segmentation by utilizing various fusion techniques. This system integrates multiple imaging modalities to provide a comprehensive and detailed analysis, enhancing the overall diagnostic process.
The primary objective of this project is to classify brain tumors and accurately segment them using a combination of different fusion techniques, including early fusion, model fusion, and PCA-based fusion. The project employs advanced machine learning models, including CNN-based and attention-based models, alongside the U-Net architecture for precise tumor segmentation.
Techniques and Implementation
- Multimodal Image Fusion:
- Early Fusion: Combines multiple imaging modalities at the input level to create a richer and more informative dataset.
- Model Fusion: Integrates the outputs of different models to leverage their strengths and improve classification accuracy.
- PCA-Based Fusion: Uses Principal Component Analysis (PCA) to reduce dimensionality and fuse features from multiple modalities effectively.
- Machine Learning Models:
- CNN-Based Models: Convolutional Neural Networks (CNNs) are used for their strong performance in image classification tasks.
- Attention-Based Models: These models focus on the most relevant parts of the input data, improving the accuracy and efficiency of tumor classification.
- Tumor Segmentation:
- U-Net Architecture: A well-known architecture for medical image segmentation, U-Net is employed to precisely segment brain tumors from the fused images.
Key Contributions
- Advanced Fusion Techniques: The project explores multiple fusion techniques, combining the strengths of early fusion, model fusion, and PCA-based fusion to enhance classification and segmentation performance.
Comprehensive Analysis: By integrating CNN-based and attention-based models, the system provides a robust and accurate analysis of brain tumors.
Precise Segmentation: The use of U-Net architecture ensures high accuracy in tumor segmentation, which is critical for effective diagnosis and treatment planning.
The project resulted into a conference publication
Publications
- Enhancing Brain Tumor Classification: A Comparative Study of Single-Model and Multi-Model Fusion Approaches. 2024 Intelligent Methods, Systems, and Applications (IMSA) DOI.
The Multimodal Image Fusion System for Brain Tumor Segmentation project represents a significant advancement in the field of medical imaging. By employing a combination of fusion techniques and advanced machine learning models, this system provides a powerful tool for the accurate classification and segmentation of brain tumors. This project highlights the potential of multimodal image fusion in improving diagnostic accuracy and patient outcomes in medical imaging.
Student List
- Mairam El-Khouli LinkedIn
Main Supervisor
items:
- Prof. Ammar Mohamed LinkedIn
Did you find this page helpful? Consider sharing it ๐